
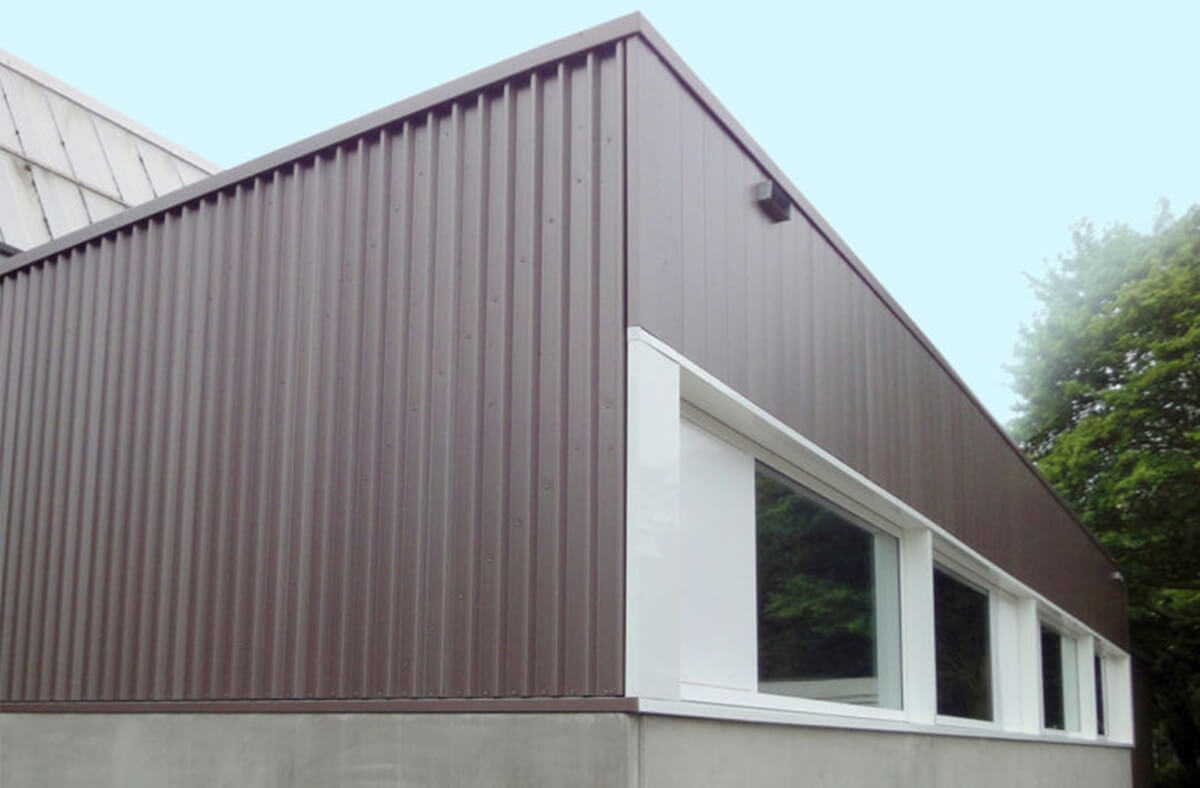

The x-ray task was the only fully novel task this year, although the other three tasks introduced modifications to keep up relevancy of the proposed challenges. In 2015, the 13th edition of ImageCLEF, four main tasks were proposed: 1) automatic concept annotation, localization and sentence description generation for general images 2) identification, multi-label classification and separation of compound figures from biomedical literature 3) clustering of x-rays from all over the body and 4) prediction of missing radiological annotations in reports of liver CT images. ImageCLEF is an ongoing initiative that promotes the evaluation of technologies for annotation, indexing and retrieval for providing information access to databases of images in various usage scenarios and domains. This paper presents an overview of the ImageCLEF 2015 evaluation campaign, an event that was organized as part of the CLEF labs 2015. We also reported the recent imageCLEF 2015 competition results that highlight the usefulness of the proposed work We validated our fully automatic technique on a set of stitched multipanel biomedical figures extracted from articles within the Open Access subset of PubMed Central repository, and achieved precision and recall of 87.16% and 83.51%, respectively, in less than 0.461 second per image, on average. It then applies a line vectorization process that con- nects prominent broken lines along the panel boundaries while eliminating insignificant line segments within the panels. The method applies local line segment detection based on the gray-level pixel changes. Since such figures may comprise images from differ- ent imaging modalities, separating them is a crucial first step for effective biomedical content-based image retrieval (CBIR): multimodal biomedical document classification and/or retrieval, for instance. We present a novel technique to separate panels from stitched multipanel figures appear- ing in biomedical research articles. To support our analysis we cross-validate model performance to reduce bias and generalization errors and perform statistical analyses to assess performance differences. In this study, we visualize the learned weights and salient network activations in a CNN based Deep Learning (DL) model to determine the image characteristics that lend themselves for improved classification with a goal of developing informed clinical question-answering systems. However, it is poorly understood how these algorithms discriminate the modalities and if there are implicit opportunities for improving visual information access applications in computational biomedicine. Researchers use novel machine learning (ML) tools to classify the medical imaging modalities.
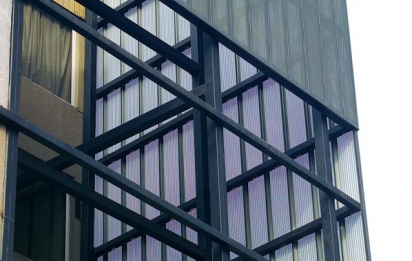
This lack of transparency is a drawback since poorly understood model behavior could adversely impact subsequent decision-making.

However, these models are perceived as black boxes since there is a lack of understanding of their learned behavior from the underlying task of interest. Convolutional neural network (CNN) has become the architecture of choice for visual recognition tasks.
